Humanized & fully human antibodies can still harbor "unhuman" motifs in their CDRs, eliciting ADAs in patients
Humanized & fully human antibodies, albeit an advancement from chimeric antibodies, can still elicit an immune response in the clinic, resulting in the production of Anti-Drug Antibodies (ADAs). This immunogenicity is primarily attributed to T-cell epitopes present in the CDRs (as shown below).
Traditional “humanness scores” cannot fully capture the immunogenicity risk in antibody CDRs. Even when such a hotspot is identified, there exists no established method to remove it with high confidence, for a single point mutation in the CDRs could abrogate antibody binding to its target. This presents a multi-objective optimization problem that is challenging to solve using traditional techniques.
A humanized antibody1 (top) and a fully human antibody2 (bottom) were found to contain T-cell epitopes (blue bars), primarily in their CDRs (green).
Reference:
1.Spindeldreher S, Karle A, Correia E, et al. T cell epitope mapping of secukinumab and ixekizumab in healthy donors[C]//MAbs. Taylor & Franci
2.Meunier S, Hamze M, Karle A, et al. Impact of human sequences in variable domains of therapeutic antibodies on the location of CD4 T-cell epitopes[J]. Cellular & Molecular Imm
AI models can predict immunogenicity in alignment with clinical data
Advances in AI have enhanced our capacity to evaluate and mitigate immunogenicity. AI models trained on vast datasets of natural antibody sequences from various species can discern subtle differences that may not be detectable using traditional sequence-identity-based algorithms.
We developed a multi-modal NLP (Natural Language Processing) model that is trained on multiple types of public and proprietary datasets. The model can predict antibody immunogenicity at both whole sequence and amino acid level. Additionally, it can assign a percentile ranking to the candidate amidst benchmark mAbs in the clinic.
We used the model to predict 217 clinical-stage antibodies with documented ADAs. The result shows good correlation (R=0.61) between predicted ADA & actual ADA.
The model can also identify the individual amino acids that are causing immunogenicity, including those in the CDRs. (Shown in Case 1)
This AI model forms the basis of our XuperHuman™ platform, which aims to identify and eliminate immunogenicity in antibodies and other protein-based therapeutics.
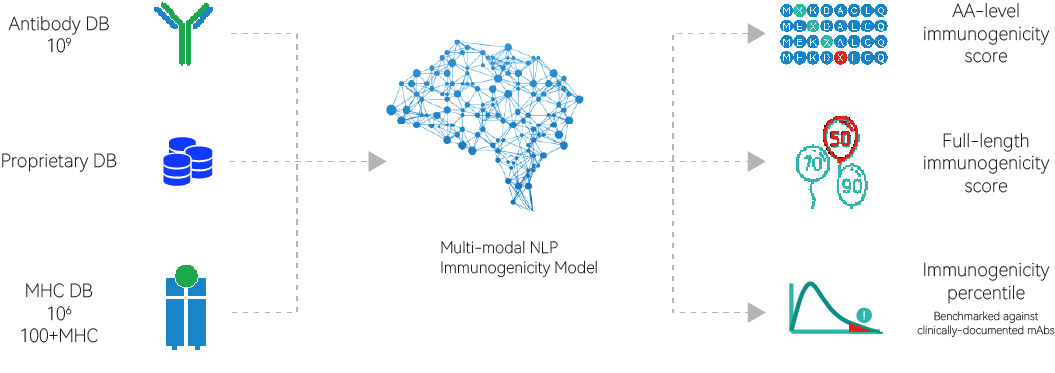
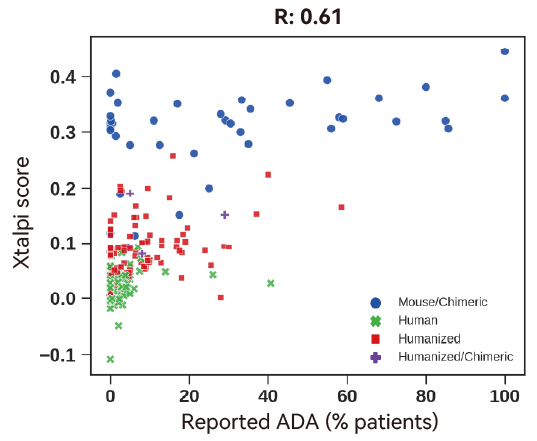